AdaMotif: Graph Simplification via Adaptive Motif Design
Hong Zhou - Shenzhen University, Shenzhen, China
Peifeng Lai - Shenzhen University, Shenzhen, China
Zhida Sun - Shenzhen University, Shenzhen, China
Xiangyuan Chen - Shenzhen University, Shenzhen, China
Yang Chen - Shenzhen University, Shen Zhen, China
Huisi Wu - Shenzhen University, Shenzhen, China
Yong WANG - Nanyang Technological University, Singapore, Singapore
Download Supplemental Material
Room: Bayshore VII
2024-10-18T13:18:00ZGMT-0600Change your timezone on the schedule page
2024-10-18T13:18:00Z
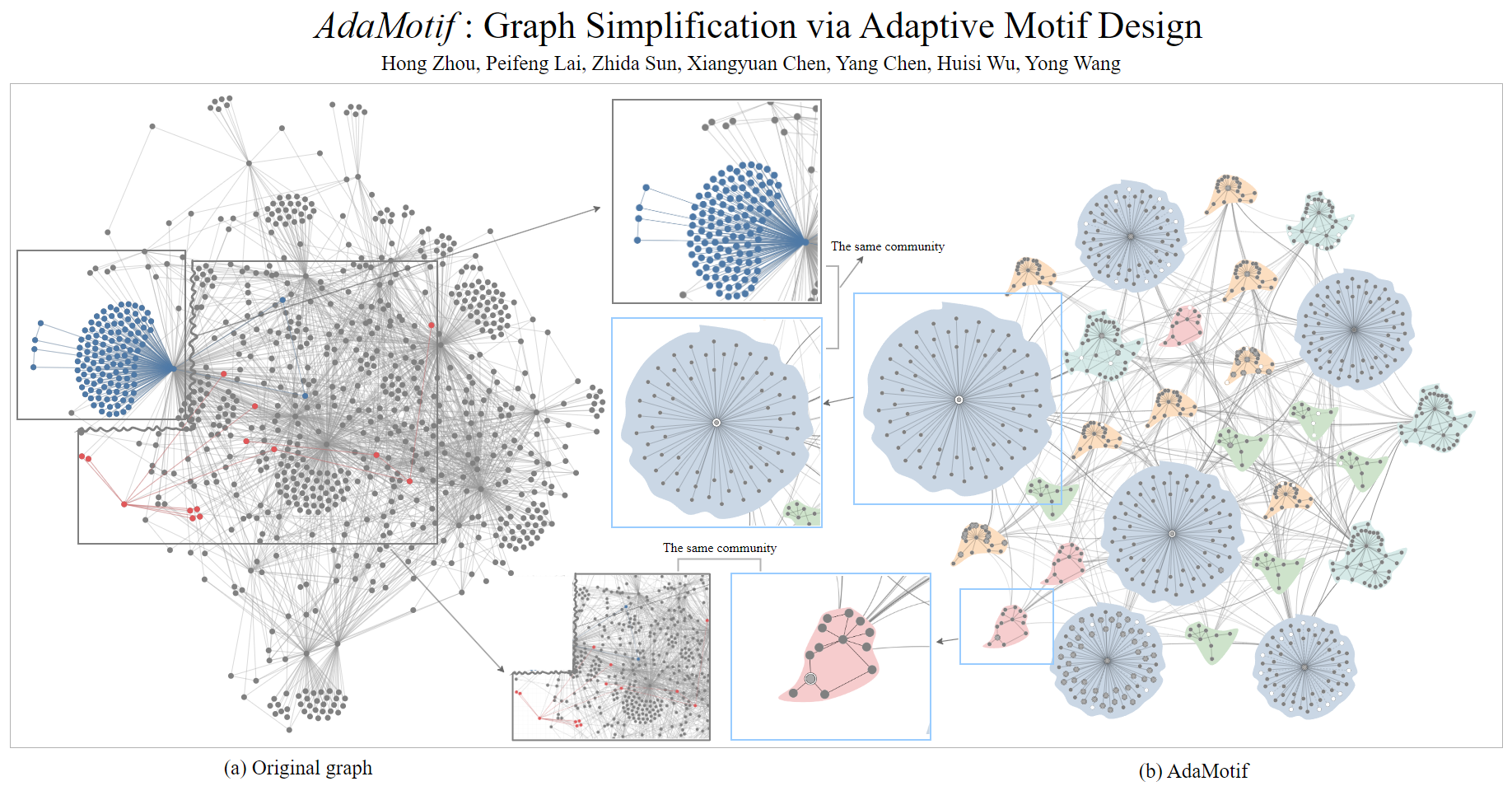
Fast forward
Full Video
Keywords
Graph visualization, node-link diagrams, graph simplification
Abstract
With the increase of graph size, it becomes difficult or even impossible to visualize graph structures clearly within the limited screen space. Consequently, it is crucial to design effective visual representations for large graphs. In this paper, we propose AdaMotif, a novel approach that can capture the essential structure patterns of large graphs and effectively reveal the overall structures via adaptive motif designs. Specifically, our approach involves partitioning a given large graph into multiple subgraphs, then clustering similar subgraphs and extracting similar structural information within each cluster. Subsequently, adaptive motifs representing each cluster are generated and utilized to replace the corresponding subgraphs, leading to a simplified visualization. Our approach aims to preserve as much information as possible from the subgraphs while simplifying the graph efficiently. Notably, our approach successfully visualizes crucial community information within a large graph. We conduct case studies and a user study using real-world graphs to validate the effectiveness of our proposed approach. The results demonstrate the capability of our approach in simplifying graphs while retaining important structural and community information.