Intuitive Design of Deep Learning Models through Visual Feedback
JunYoung Choi - VIENCE Inc., Seoul, Korea, Republic of. Korea University, Seoul, Korea, Republic of
Sohee Park - VIENCE Inc., Seoul, Korea, Republic of
GaYeon Koh - Korea University, Seoul, Korea, Republic of
Youngseo Kim - VIENCE Inc., Seoul, Korea, Republic of
Won-Ki Jeong - VIENCE Inc., Seoul, Korea, Republic of. Korea University, Seoul, Korea, Republic of
Screen-reader Accessible PDF
Download Supplemental Material
Room: Bayshore VI
2024-10-17T18:21:00ZGMT-0600Change your timezone on the schedule page
2024-10-17T18:21:00Z
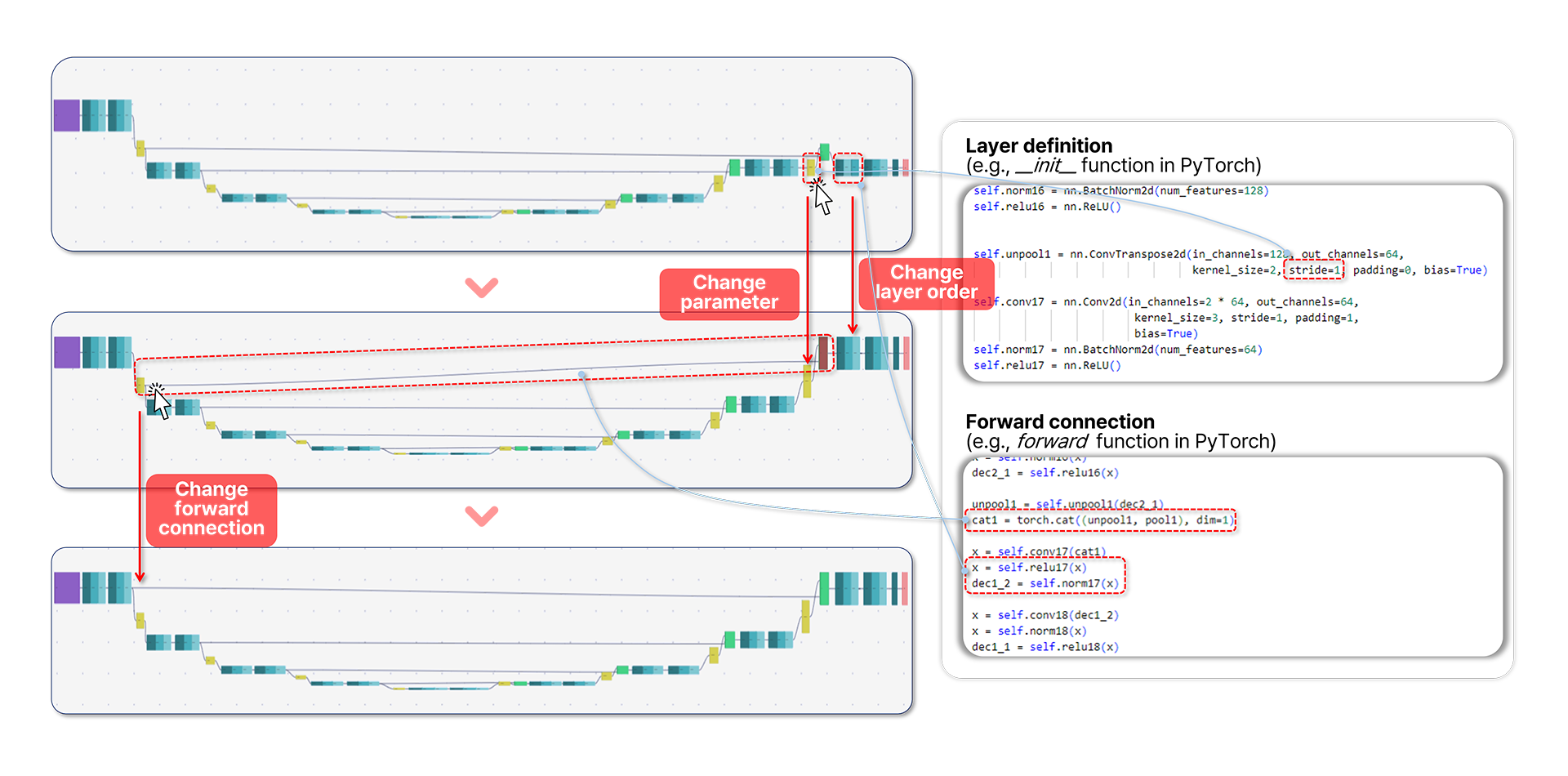
Fast forward
Full Video
Keywords
Deep learning, visual programming, explainable AI.
Abstract
In the rapidly evolving field of deep learning, traditional methodologies for designing models predominantly rely on code-based frameworks. While these approaches provide flexibility, they create a significant barrier to entry for non-experts and obscure the immediate impact of architectural decisions on model performance. In response to this challenge, recent no-code approaches have been developed with the aim of enabling easy model development through graphical interfaces. However, both traditional and no-code methodologies share a common limitation that the inability to predict model outcomes or identify issues without executing the model. To address this limitation, we introduce an intuitive visual feedback-based no-code approach to visualize and analyze deep learning models during the design phase. This approach utilizes dataflow-based visual programming with dynamic visual encoding of model architecture. A user study was conducted with deep learning developers to demonstrate the effectiveness of our approach in enhancing the model design process, improving model understanding, and facilitating a more intuitive development experience. The findings of this study suggest that real-time architectural visualization significantly contributes to more efficient model development and a deeper understanding of model behaviors.