FAVis: Visual Analytics of Factor Analysis for Psychological Research
Yikai Lu - University of Notre Dame, Notre Dame, United States. University of Notre Dame, Notre Dame, United States
Chaoli Wang - University of Notre Dame, Notre Dame, United States
Screen-reader Accessible PDF
Download preprint PDF
Room: Bayshore VI
2024-10-17T16:00:00ZGMT-0600Change your timezone on the schedule page
2024-10-17T16:00:00Z
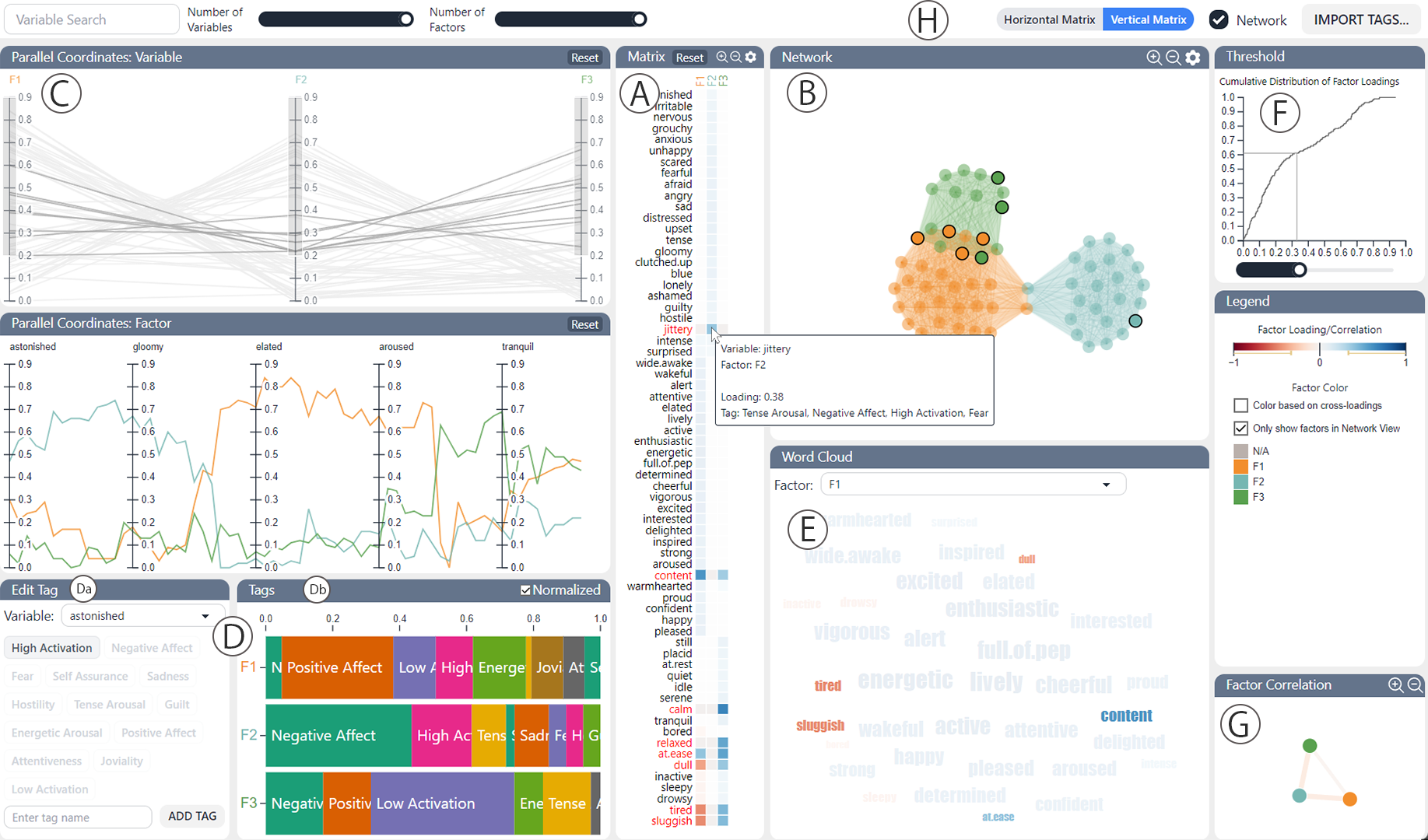
Fast forward
Full Video
Keywords
Machine Learning, Statistics, Modelling, and Simulation Applications, Coordinated and Multiple Views, High-dimensional Data
Abstract
Psychological research often involves understanding psychological constructs through conducting factor analysis on data collected by a questionnaire, which can comprise hundreds of questions. Without interactive systems for interpreting factor models, researchers are frequently exposed to subjectivity, potentially leading to misinterpretations or overlooked crucial information. This paper introduces FAVis, a novel interactive visualization tool designed to aid researchers in interpreting and evaluating factor analysis results. FAVis enhances the understanding of relationships between variables and factors by supporting multiple views for visualizing factor loadings and correlations, allowing users to analyze information from various perspectives. The primary feature of FAVis is to enable users to set optimal thresholds for factor loadings to balance clarity and information retention. FAVis also allows users to assign tags to variables, enhancing the understanding of factors by linking them to their associated psychological constructs. Our user study demonstrates the utility of FAVis in various tasks.