KD-INR: Time-Varying Volumetric Data Compression via Knowledge Distillation-based Implicit Neural Representation
Jun Han -
Hao Zheng -
Change Bi -
Screen-reader Accessible PDF
DOI: 10.1109/TVCG.2023.3345373
Room: Bayshore I
2024-10-16T12:30:00ZGMT-0600Change your timezone on the schedule page
2024-10-16T12:30:00Z
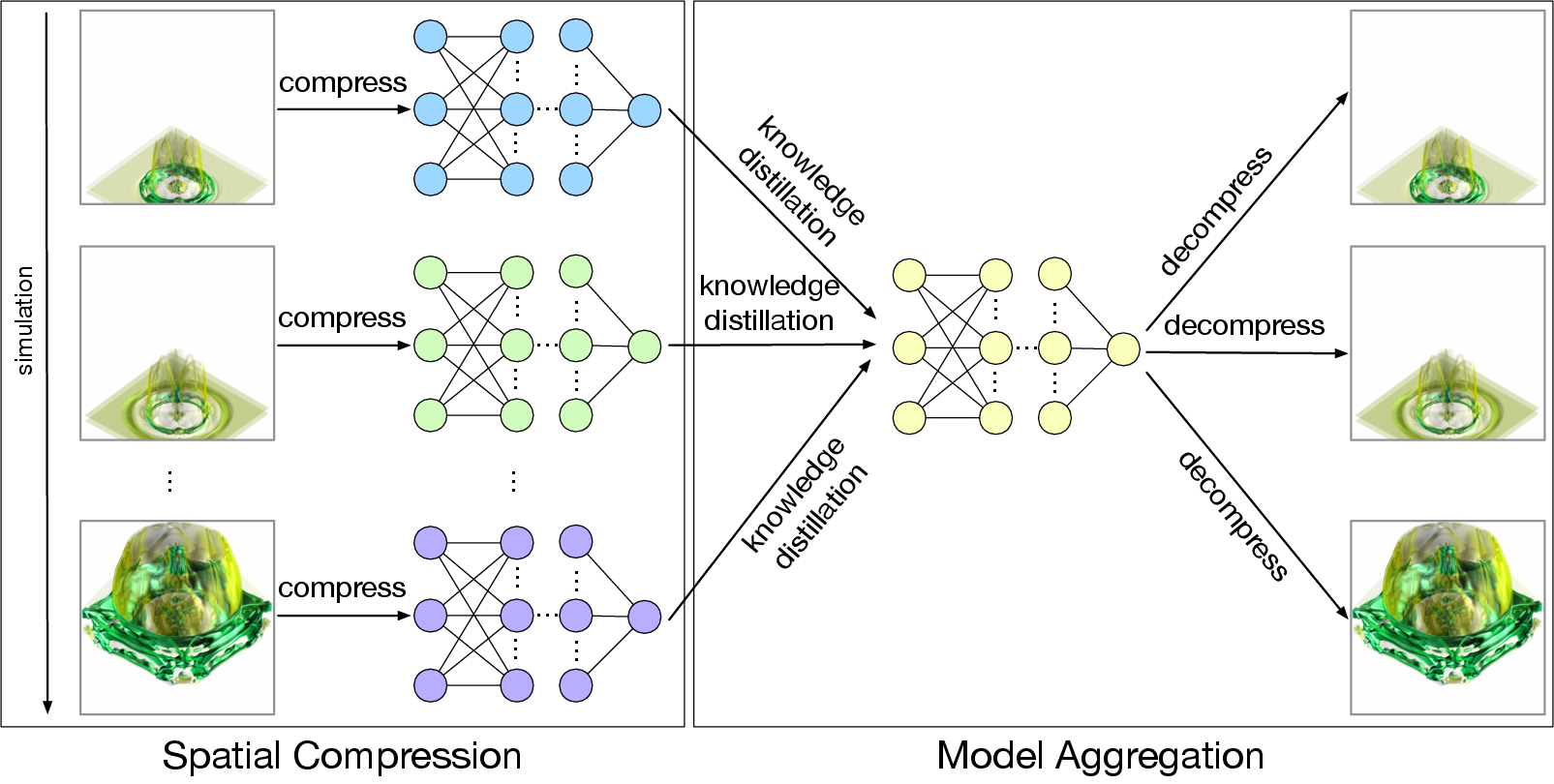
Fast forward
Full Video
Keywords
Time-varying data compression, implicit neural representation, knowledge distillation, volume visualization.
Abstract
Traditional deep learning algorithms assume that all data is available during training, which presents challenges when handling large-scale time-varying data. To address this issue, we propose a data reduction pipeline called knowledge distillation-based implicit neural representation (KD-INR) for compressing large-scale time-varying data. The approach consists of two stages: spatial compression and model aggregation. In the first stage, each time step is compressed using an implicit neural representation with bottleneck layers and features of interest preservation-based sampling. In the second stage, we utilize an offline knowledge distillation algorithm to extract knowledge from the trained models and aggregate it into a single model. We evaluated our approach on a variety of time-varying volumetric data sets. Both quantitative and qualitative results, such as PSNR, LPIPS, and rendered images, demonstrate that KD-INR surpasses the state-of-the-art approaches, including learning-based (i.e., CoordNet, NeurComp, and SIREN) and lossy compression (i.e., SZ3, ZFP, and TTHRESH) methods, at various compression ratios ranging from hundreds to ten thousand.